#raytracing


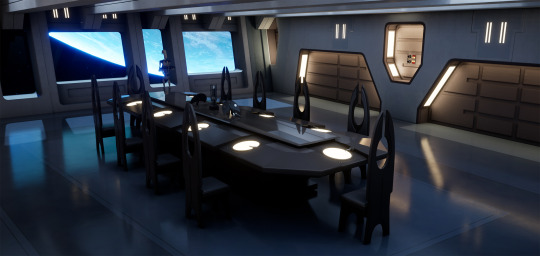


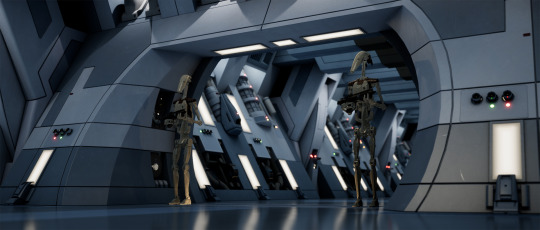



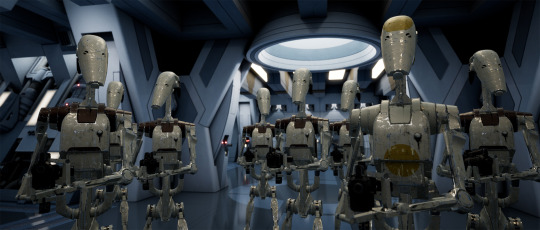

UE4 - Star Wars: Droid Control Ship - by Jorge Barros(myself)
I’m so very thrilled to finally be able to share my greatest personal project so far, a tribute to a beloved legendary saga, born from the brilliant mind of George Lucas. This is a playable real-time environment recreated after the first scenes in Star Wars - Episode I, inside the Trade Federation’s Droid Control Ship, that I started a few years ago, but have only been able to make progress on, on and off, during free time, and through a lot of lightmap desperation!..
Everything was made by me alone, apart from all sound used in the videos.
[Cinematic video]
[Gameplay video]
Check [my ArtStation] for high resolution images and some 360º panoramas!
Imagine you had a function P that upon swallowing a subset E of a universal set Ω will return a number x from the real number line. Keep imagining that P must also obey the following rules:
- IfP can eat the subset, it will always return a nonnegative number.
- If you give P the universe Ω, it will give you back 1.
- If you collected together disjoint subsets and gave them to P to process, the result would be the same as feeding P each subset individually and adding the answers.
Simple, if odd out of context.
Mathematicians have a curious way of pulling magic of out simplicity.
~
Probability today is studied as a mathematical science based on the three axioms (flavored by set theory) stated above. These are the “first principles” from which many other, derivative propositions have been speculated and proved. The results of the modern study of probability fuel many branches of engineering, including signals processing in electrical and computer engineering, the insurance and finance industries, which translate probabilities into economic movement, and many other enterprises. Along the way it borrowed from the other giants of mathematics, analysis and algebra, and goes on generating new research ideas for itself and other fields. This is the way of math: set down a bunch of rules (preferably simple to start) and see how their consequences play out.
But what is probability? If it is quantitative measure, what is it measuring? How valid is that measure and how could it be checked? Even these are rich questions to probe. A working qualitative description for practitioners might be that probability quantifies uncertainty. It answers with some degree of success such questions as “What is the chance?” or “How likely is this?” If a system contains uncertainty, probability provides the model for handling it, and data gathered from the system can validate or improve the probability model.
According to Wikipedia, there are three main interpretations for probability:
- Frequentists talk about probabilities only when dealing with experiments that are randomandwell-defined. The probability of a random event denotes the relative frequency of occurrence of an experiment’s outcome, when repeating the experiment. Frequentists consider probability to be the relative frequency “in the long run” of outcomes.
- Subjectivists assign numbers per subjective probability, i.e., as a degree of belief.
- Bayesians include expert knowledge as well as experimental data to produce probabilities. The expert knowledge is represented by a prior probability distribution. The data is incorporated in a likelihood function. The product of the prior and the likelihood, normalized, results in a posterior probability distribution that incorporates all the information known to date.
~
So let’s reinterpret the math.
Let Ω be the sample space, the set of all possible outcomes, be Ei be subsets of Ω which denote different events for different i, and be the set of all events. Then a probability map P is defined as any function from → ℝsatisfying
- P(Ei) ≥ 0
All probabilities are non-negative. - P(Ω) = 1
It is certain that one of the outcomes of Ω will happen. - Ei ∩ Ej = ∅ if i≠j ⇔ P(∑iEi) = ∑iP(Ei)
Probabilities of disjoint events can be added to get the probability of any of them happening.
–
Image generated by Rene Schwietzke using POV-Ray, a raytracing freeware that creates 3D computer graphics.
Further reading:
A First Course in Probability (8th ed., 2010), Sheldon Ross.
Probability and Statistics (4th ed., 2010), Mark J. Schervish and Morris H. Degroot.
Post link
